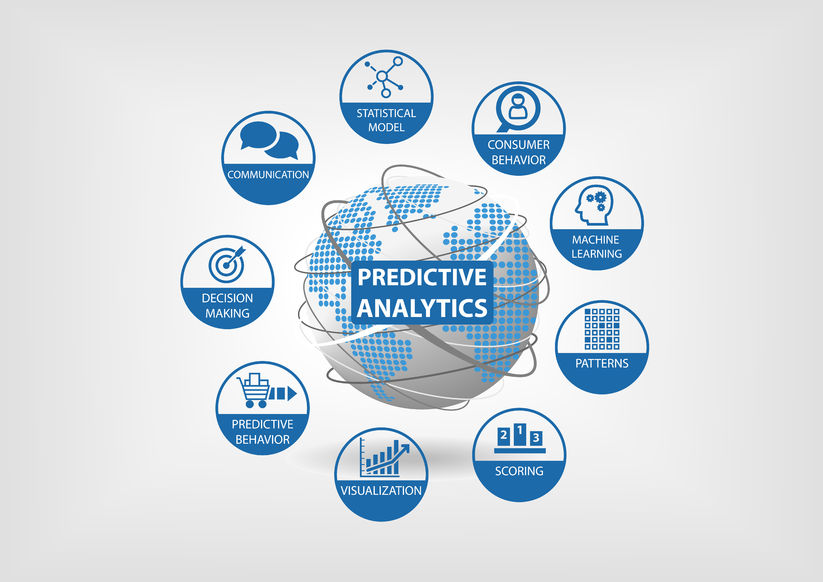
25 Sep How Artificial Intelligence and Predictive Analytics Can Help You Reduce Customer Churn
Real-world use cases that demonstrate how artificial intelligence can help you gauge customer sentiment and which customers are at risk for cancellation are here. But, it isn’t that easy. You have to be already gathering customer data in a relational database.
In addition to good data, you’ll need someone with a keen eye for your business and industry to best use that information to prevent churn from happening in the future at your company. Someone that can identify whether it’s people, processes or your product that needs some TLC.
If you don’t have relational data or someone to analyze it, you’re not alone. But, you’ll need to get there to remain competitive in today’s customer service era. We’ll also discuss what you’ll need to implement to ensure that you have the right data in the future to begin analyzing customer sentiment or predict customer churn.
Analyze Customer Reviews to Gauge Sentiment
For companies large or small, it can be difficult to gauge how prospects and customers feel about your brand, especially if your products are sold by hundreds of retailers. Opinions shared on social media and review sites might be in the thousands daily.
In fact, according to Pew Research Center, “Nearly six-in-ten adults (58%) have done research online about the products and services they buy, and about a quarter (24%) have posted comments or reviews online about the things they buy.”
Many companies use social media monitoring tools to find and review one-by-one brand mentions, but what those tools don’t tell you is how do consumers feel about your brand. From a high level, many companies or brands are wondering – do people feel more positive or negative about my brand or company?
One company – Revuze.it – is aiming to help companies with this problem of gauging brand sentiment by applying NLP (Natural Language Processing) to scan a review in its entirety. In many cases, many companies only review their status in a rating system, for example, as seen on amazon.com.
Revuze’s software utilizes NLP, a form of artificial intelligence, and computational linguistics to filter for words that reveal customer attitudes and emotion, which are revealed in a sentiment analysis report. Their software is able to scan social media, surveys, emails (these are your own with your customers), transcripts, call center notes and the web.
Interestingly, Revuze was named by CB Insights as an “early stage Israeli B2B Software Company to Watch.” CB Insights used their own AI-powered software, Mosaic, which uses public data and predictive algorithms to provide intelligence on private companies, to compile the list.
While we couldn’t locate any reviews online of Revuze, it appears that Neutrogena successfully used Revuze to “analyze online ratings and reviews in conjunction with Bazaarvoice, which captures and syndicates them,” according to an article from AdAge on the Neutrogena campaign.
Artificial Intelligence Can Help Predict Customer Churn
Because every marketer and CEO has repeatedly heard that it’s less expensive to keep current customers than to acquire new ones, it’s important to track why your customers are leaving. So, if you’re not doing that, you should be.
More importantly, what if you could identify customers that are at risk of leaving so that you could work to salvage the relationship before they leave you? AI has successfully been implemented in this scenario as well when combined with data sets.
In fact, Kissmetrics says the process includes only three steps. Although it sounds easy,it’s much more complex than that for most companies.
First, you must be already gathering customer data. You should have all the standard data, such as customer location, age, email, title, etc…, but you should also be inquiring why customers are leaving when they leave. It’s important to record this information too.
The second challenge lies in whether it’s structured or unstructured data. Structured data is highly organized and readily searchable within a relational database, like a CRM. Unstructured data doesn’t often live in a database, but in a silo without any organizational structure to it. But, if you’re lucky enough to be that organized and have structured data, the process works like this:
- Gather customer historical data and save to a .csv file.
- Upload that data to a prediction service, like BigML, that can automatically create a predictive model.
- Apply that model to each current customer to help determine whether they’re at risk in leaving you.
Now, get your best knowledge worker, in this case a data scientist, that understands your business and the industry it works within, and put a response team in place to prevent at-risk customers from leaving. Additionally, if any obvious holes in customer service are to blame, put processes in place to prevent those from happening further.
Summary
The clear lesson here is that if you aren’t gathering substantial data on prospects and customers, you should be. Put a CRM in place and more importantly, have an expert set it up correctly. Too many companies fall short here and don’t use such systems properly to their advantage or the way they were designed to be used.
And, finally – not only should you always be closing, but you should always be analyzing. Check that the system is being used as it should be and the data you’re trusting others to enter is accurate and complete. Analyze your data and data-gathering tools. Now, you’re on the right track to begin using artificial intelligence-based predictive analytics.